How the Brain Recognizes What the Eyes See
(Originally published by the Salk Institute)
June 8, 2017
If you think self-driving cars can’t get here soon enough, you’re not alone. But programming computers to recognize objects is very technically challenging, especially since scientists don’t fully understand how our own brains do it.
Now, Salk Institute researchers have analyzed how neurons in a critical part of the brain, called V2, respond to natural scenes, providing a better understanding of vision processing. The work is described in Nature Communications on June 8, 2017.
“Understanding how the brain recognizes visual objects is important not only for the sake of vision, but also because it provides a window on how the brain works in general,” says Tatyana Sharpee, an associate professor in Salk’s Computational Neurobiology Laboratory and senior author of the paper. “Much of our brain is composed of a repeated computational unit, called a cortical column. In vision especially we can control inputs to the brain with exquisite precision, which makes it possible to quantitatively analyze how signals are transformed in the brain.”
Although we often take the ability to see for granted, this ability derives from sets of complex mathematical transformations that we are not yet able to reproduce in a computer, according to Sharpee. In fact, more than a third of our brain is devoted exclusively to the task of parsing visual scenes.
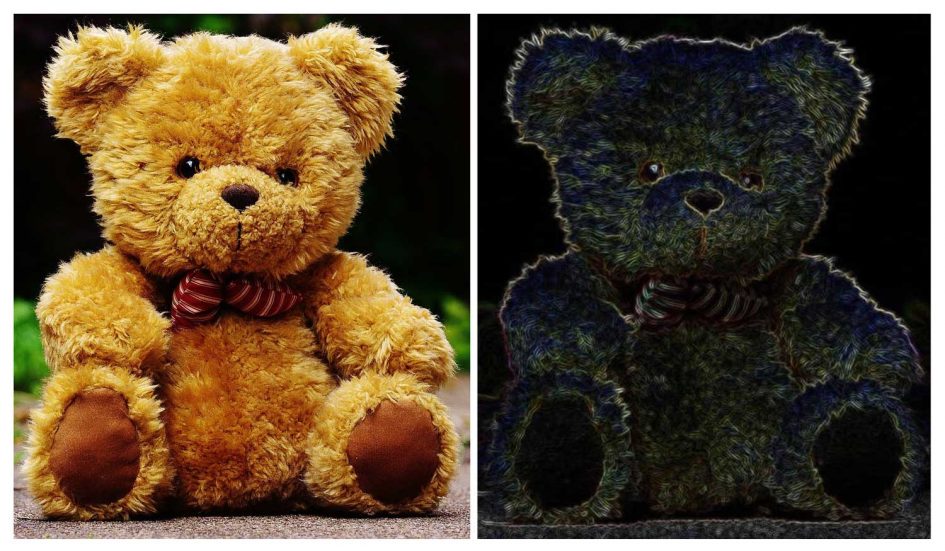
Our visual perception starts in the eye with light and dark pixels. These signals are sent to the back of the brain to an area called V1 where they are transformed to correspond to edges in the visual scenes. Somehow, as a result of several subsequent transformations of this information, we then can recognize faces, cars and other objects and whether they are moving. How precisely this recognition happens is still a mystery, in part because neurons that encode objects respond in complicated ways.
Now, Sharpee and Ryan Rowekamp, a postdoctoral research associate in Sharpee’s group, have developed a statistical method that takes these complex responses and describes them in interpretable ways, which could be used to help decode vision for computer-simulated vision. To develop their model, the team used publicly available data showing brain responses of primates watching movies of natural scenes (such as forest landscapes) from the Collaborative Research in Computational Neuroscience (CRCNS) database.
“We applied our new statistical technique in order to figure out what features in the movie were causing V2 neurons to change their responses,” says Rowekamp. “Interestingly, we found that V2 neurons were responding to combinations of edges.”
The team revealed that V2 neurons process visual information according to three principles: first, they combine edges that have similar orientations, increasing robustness of perception to small changes in the position of curves that form object boundaries. Second, if a neuron is activated by an edge of a particular orientation and position, then the orientation 90 degrees from that will be suppressive at the same location, a combination termed “cross-orientation suppression.” These cross-oriented edge combinations are assembled in various ways to allow us to detect various visual shapes. The team found that cross-orientation was essential for accurate shape detection. The third principle is that relevant patterns are repeated in space in ways that can help perceive textured surfaces of trees or water and boundaries between them, as in impressionist paintings.
The researchers incorporated the three organizing principles into a model they named the Quadratic Convolutional model, which can be applied to other sets of experimental data. Visual processing is likely to be similar to how the brain processes smells, touch or sounds, the researchers say, so the work could elucidate processing of data from these areas as well.
“Models I had worked on before this weren’t entirely compatible with the data, or weren’t cleanly compatible,” says Rowekamp. “So it was really satisfying when the idea of combining edge recognition with sensitivity to texture started to pay off as a tool to analyze and understand complex visual data.”
But the more immediate application might be to improve object-recognition algorithms for self-driving cars or other robotic devices. “It seems that every time we add elements of computation that are found in the brain to computer-vision algorithms, their performance improves,” says Sharpee.
The work was funded by the National Science Foundation.