Mathematics Drops Clues About the Brain and Mind
by Sally Johnson
Sophisticated math and engineering can help advance our understanding of the brain
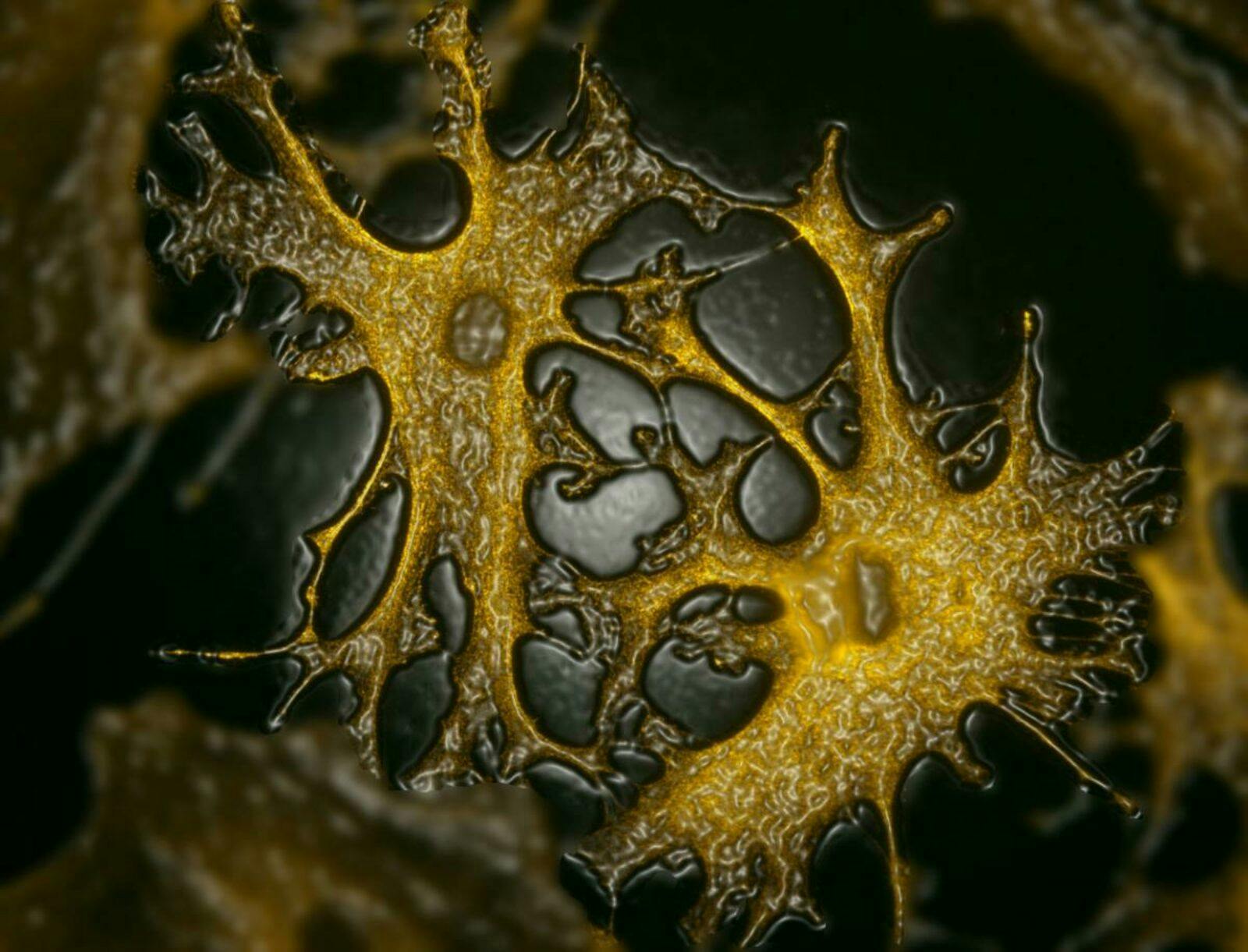
The Author
The Researcher
Human brains are incredibly complex computational systems, with trillions of interconnected cells and billions of neurons forming a huge network. How our brains work and communicate—with the ability to perceive emergent properties such as self-awareness and consciousness—remains largely an elusive mystery.
Gabriel A. Silva, associate director of the Kavli Institute for Brain and Mind, as well as a professor of bioengineering and neurosciences at UC San Diego, and director of the Center for Engineered Natural Intelligence, is studying how the brain computes and processes information—by approaching it from a systems engineering and mathematics perspective.
“I simply don’t think we’re going to understand how the brain works as a coherent structure without bringing in engineering and math,” says Silva.
“I’m convinced there are entire areas of mathematics and applied engineering that have the opportunity to make major contributions to our understanding of the brain but we just haven’t connected the dots yet.”
He views mathematics as a unifying language and framework to describe and keep track of all the moving parts and details that we know about the brain.
And it appears he’s onto something. After a mathematical prediction made by a theoretical framework suggested there’s a design constraint behind the convoluted shape of neurons, Silva went hunting for it. He and colleagues discovered that real neurons seem to optimize their shape to a refraction ratio, which is a balance between how quickly information propagates within a neuron or network and how much time cells take to process and react to it.
“It’s really cool because it was a discovery about real biological neurons that wasn’t serendipitous at all—the math pointed us in that direction,” Silva says.
So Silva and colleagues at UC San Diego, along with collaborators at Microsoft and the Kavli Neuroscience Discovery Institute at Johns Hopkins University, are developing methods to apply that same design constraint to analyze brain organoids of autistic patients in an experiment parallel to a clinical trial. Organoids are essentially a tiny model of your brain in a dish, which can be created via cells from a cheek swab or skin biopsy. These cells exhibit your unique developmental genetic program, he explains, or at least certain aspects of it.
They will explore a hypothesis that a mismatch in the refraction ratio can cause a breakdown in neuron and network signaling in autistic individuals. “We’re throwing some really sophisticated mathematics at the analysis of organoid data networks,” Silva says. “It’s a form of personalized neuroscience, and one day it might be possible to predict how you will clinically respond to drugs before they’re prescribed.”
Beyond this, Silva is also interesting in exploring the mind, one of the most elusive and least understood parts of the brain. He’s intrigued by the concept of emergence: How do neurons that form networks or brain regions and their interactions translate into emergent complex behavior?
“At the whole person level, we’re just starting to explore how some of these engineering ideas that apply at network scales translate to emergence,” says Silva.
“I’m really interested in how aspects of the mind come out of, emerge from the structural pieces that make up the brain.”
To attempt to understand this, the perspective Silva and colleagues bring to the table is: Can we understand those emergent aspects of what makes up the brain from the wetware that makes up the brain by bringing mathematical and engineering tools to bear? “We’re just starting to think about it in a serious way,” he says. “It’s the link to the emergent properties of what the mind is. Half the time we don’t know where this will take us. We’re following our intuition at the fringe of knowledge.”
And in the near future of neuroengineering, Silva also expects to see a convergence of nanotechnology, brain machine interfaces, and artificial intelligence.
“In particular, we’ll see progress on neural prostheses,” Silva says. “There’s an opportunity here to create smart and adaptive brain interfaces. Today’s state-of-the-art neural prosthesis, which gets implanted into a patient, has varying degrees of clinical success. They have sophisticated engineering, but once it’s put into a patient it becomes a static piece of engineering. In other words, its design and functionality are fixed.”
The next evolutionary step is to be able to change their functional outputs and learn from the needs of the patient. Onboard machine learning within these devices or the cloud will allow communication with the patient’s device to adapt their functionality.
“If the intent of the device is to electrically stimulate part of the brain, being able to modulate in an adaptive way as the disease progresses is important. Or frankly, just to account for differences between individuals who have the same disorder,” Silva adds. “This has tremendous potential—and it’s also being driven, in part, by the gaming industry to create really immersive and responsive games.”
This can also help autistic children learn motor and coping skills, Silva points out. “Beyond entertainment, there are many practical applications for this type of technology,” he says. “We’re just starting to scratch the surface of applying really sophisticated machine learning to the design of brain interfaces.”