Translating Birdsong
by Sally Johnson
Timothy Gentner decodes birds’ neural activity into song
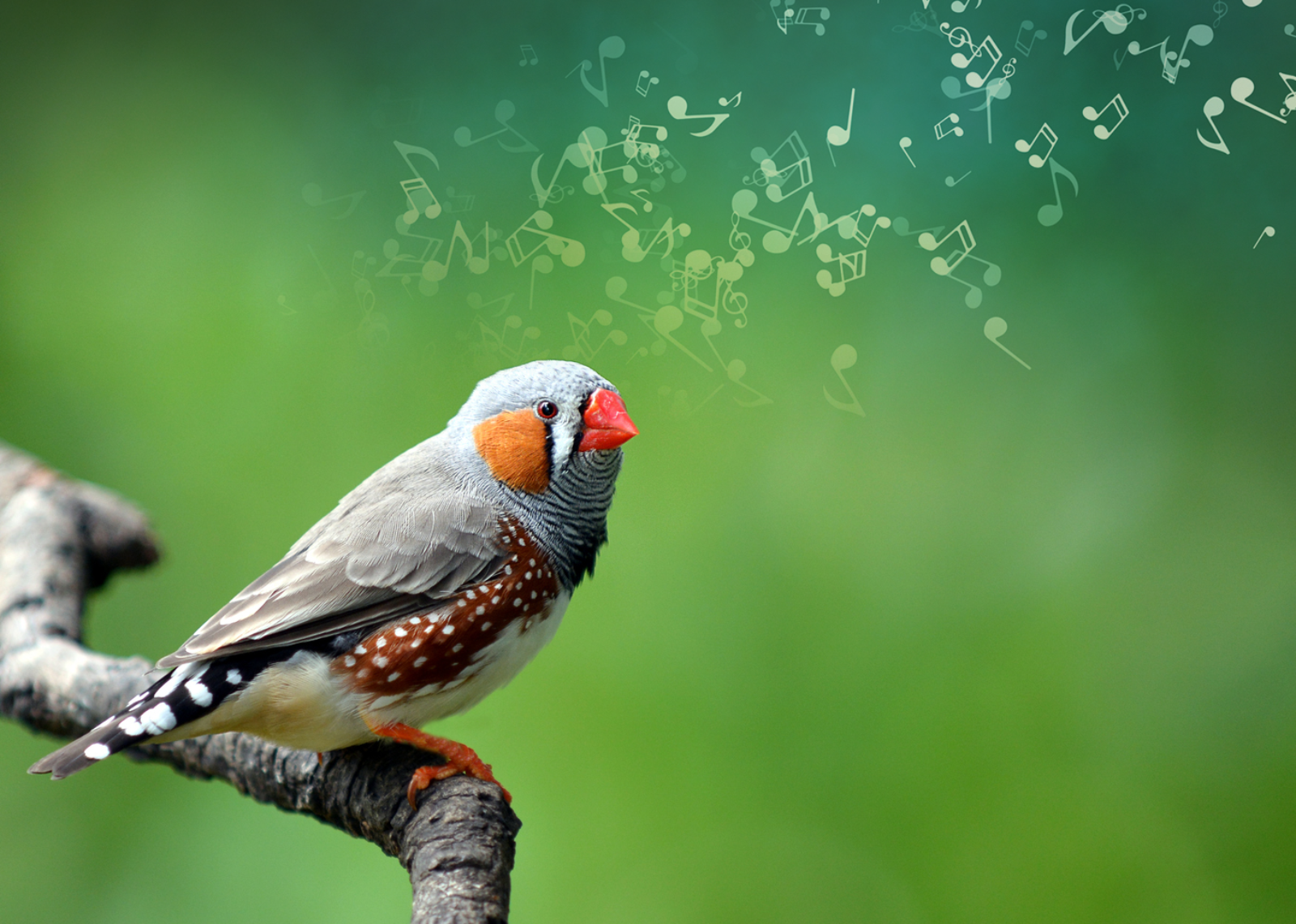
The Author
The Researcher
Timothy Gentner’s Auditory Neuroscience lab recently collaborated with Vikash Gilja’s Electrical and Computer Engineering lab, and others at UC San Diego, to decode a songbird’s brain activity to produce its song.
Now, they hope to apply lessons learned from songbirds directly to humans to create an entirely new type of speech prosthesis to allow users to speak freely—extending beyond the rudimentary communication abilities of current technologies.
“We’re talking about, potentially, a fundamentally different technology to restore communication,” says Gentner, whose fascination with animal communication began while volunteering at the Woodland Park Zoo in Seattle as an undergraduate student.
Gentner was intrigued by siamangs, large gibbons that live in trees and “sing incredible duets.” So he started recording them to try to decode their songs, and went on to study animal communication and neural mechanisms. But instead of primates, he focuses on songbirds.
Bird song is a very well developed field, which centers on attempting to understand the neurobiology and behavioral ecology of song, song production, and song learning.
“Bird song is one of the premiere neurobiological model organisms for vocal learning,” says Gentner. “Only a few groups of animals feature complex, learned vocal behaviors—songbirds, humans, whales and dolphins, and a few others.”
Zebra finches are the dominant model species in songbird research because their vocalization is highly repeatable and shares some similarities with human speech. “They sing a structured song many times throughout the day, so we can record complex, learned neural activity,” he explains.
To do this, silicon electrodes are implanted into male adult zebra finches. As they sing, the electrical activity of several populations of neurons within the sensorimotor area of their brain—involved in controlling the muscles responsible for singing—get recorded.
Then the challenge is to decode it in a way that can be used to generate the vocal signal itself.
This is where machine learning comes in. “We couldn’t do this without machine learning,” says Gentner. “We’re essentially placing antennas into a bird’s brain and measuring little voltage traces. Machine learning takes what looks like complex noise with spikes and crackles in it and figures out how to decode it.”
This enables transforming the measurements into the variables that drive their model of the zebra finch’s vocal organ, the syrinx. The syrinx in birds works like the larynx in humans, but with slight modifications. It has a group of muscles controlling airflow and tension, similar to vocal cords, which are responsible for how fast they vibrate.
“We’re trying to map neural activity so we can figure out how the math works and how the engineering has to work to get enough data to enable producing not just a fixed library of words, if you will, but all of the possible syllables that can be produced and combined in any way you want,” Gentner says.
One of the main lessons learned from zebra finches is that different regions of the brain appear to be contributing to the structure of brain signals, which introduces another challenge of understanding these really complex behaviors.
“We can describe them in really simple terms or zero-in on the fine level of detail required for coordinating all of the muscle groups that need to work together and to integrate the sensory-motor feedback required to modify the muscles,” he elaborates. “Complex behavior is a range of different components working together across time and spatial scales—and the brain controls all of it.”
Another lesson learned is that recording collective activity across groups of neurons, “works surprisingly well in driving some of our models,” Gentner says. “It gives us clues about the way neural systems are structured, as well as their redundancy and resilience. Individual neurons jump into and out of circuitry, and yet the bird is still able to produce the behavior. Whether we’re recording from one neuron or a group of them, we can still extract the same kind of information.”
Instead of trying to recreate sound, the researchers wondered what if they tried to recreate the components of a model that described the way the vocal apparatus, the syrinx, works in birds?
So rather than trying to map the neural activity to the song, they mapped the neural activity to the dynamics of the model of the syrinx. And once they figured out how the variables in the model and equation are controlled by neural activity, they could just let the model run and it generates the song—right down to the pitch, volume, and timbre of the original.
Gentner and his UCSD colleagues are now working to apply what they’re learning from birds directly to humans. This means implanting humans with electrical arrays, which, as you can imagine, requires numerous checks and balances.
It’s not entirely clear yet when a speech prosthesis based on this work might be available, but the group hopes to begin piloting studies in humans within the next two years.
Gentner notes that Kavli Institute for Brain and Mind (KIBM) was instrumental to this work. “We had a crazy idea, and KIBM helped fund it,” he says. “We couldn’t have done this without them and their support for high-risk work with the potential to make a big impact down the road.”
What’s next? Gentner and colleagues are already working with European starlings, which produce a more flexible range of sounds and can mimic all sorts of noises. European starlings haven’t been studied as much as zebra finches because their song is a lot more complex and variable, “so it’s the next level of challenge for us,” he says.