Translating Brain Activity Into Speech
by Sally Johnson
Brain-computer interface allows neural signals to be decoded into speech
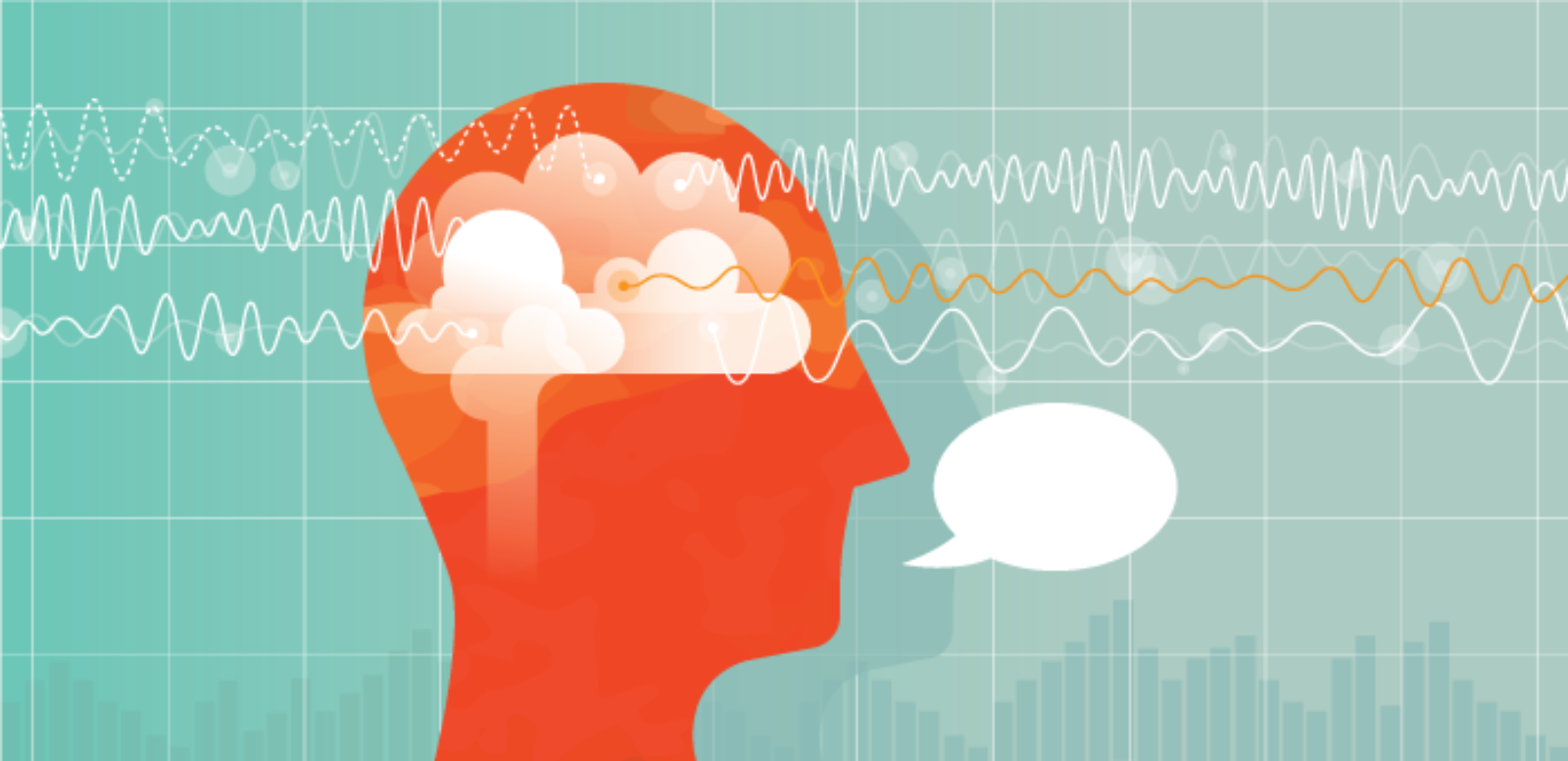
The Author
The Researcher
Brain-computer interfaces (BCIs) have come a long way during the past 10 years. And Edward Chang, a professor of neurological surgery, as well as a member of the Kavli Institute of Fundamental Neuroscience at the University of California, San Francisco (UCSF), has taken it a giant step further by decoding speech directly from brain activity.
This work is incredibly promising and offers hope for many patients, including those with speech loss due to paralysis, who are currently limited to communicating by spelling words one letter at a time with eye movements or muscle twitches.
Previous work has made important progress, but there is still a long way to getting communication fast and accurate enough for practical application in paralyzed people. Current technologies are limited to about 10 words per minute, whereas fluent speech is around 150 word per minutes.
Chang was inspired to search for a better way to translate brain activity into speech “by treating people after serious brain injuries and feeling helpless that we don’t have better ways to restore someone’s ability to walk or talk,” he says.
So he and his group “are working hard to develop a speech neuroprosthetic device designed to translate brain activity into words and sentences—primarily for people who are paralyzed and can’t communicate,” Chang says.
To do this, Chang and colleagues worked with four epilepsy patients who had special electrodes placed on their brains for clinical indications for both localizing seizures and mapping out and protecting the speech centers.
These patients were asked to read sentences aloud and, as they did, the device’s electrodes recorded the activity within their speech centers. This information was then fed to a neural network to detect any patterns associated with the words being spoken.
The researchers translate brain activity via machine-learning techniques with “recurrent neural networks,” similar to state-of-the-art speech recognition and language translation algorithms. Recurrent neural networks are a class of artificial networks where connections between nodes form a directed graph along a temporal sequence, which allows it to display temporal dynamic behavior. “Recurrent neural networks are especially good at modeling the context of preceding information related to speech,” Chang says.
While the patients spoke, the neural network analyzed their brain activity and predicted which words and sentences were spoken. For one of the participants, it showed 97% accuracy—demonstrating that the neural network was able to translate brain activity into written words and sentences that look like natural speech. This is significant because it marks the first time this has been achieved in real time with this level of accuracy and in actual sentences.
Our machine-learning algorithms decode brain activity to text, or movements of the vocal tract that subsequently generate synthesized speech sounds. While there’s much to still do, I’m constantly surprised by how much progress has been made within the field during the past decade.”
Edward Chang
BCI technology allows Chang’s group to integrate engineering with neuroscience, with a practical goal of restoring neurological function. “While we don’t have cures for many severe neurological diseases yet, the potential application of BCI technology has promise to improve quality of life for many suffering people,” he says.
Part of this project received funding from Facebook, which in 2017 unveiled its goal of creating a wearable, noninvasive device to translate neural signals to text.
Chang envisions many applications for BCIs ahead. “The need for better technology to assist people who are injured is massive, and we’re learning how to harness it in much more directed and powerful ways,” he says. “I’m looking forward to a future where we can make meaningful impact on quality of life for people suffering from neurological diseases.”