Watching Neuronal Connections Take Shape in the Brain
by Alla Katsnelson
Researchers at the Kavli Neuroscience Discovery Institute combine state of the art in vivo imaging with machine learning
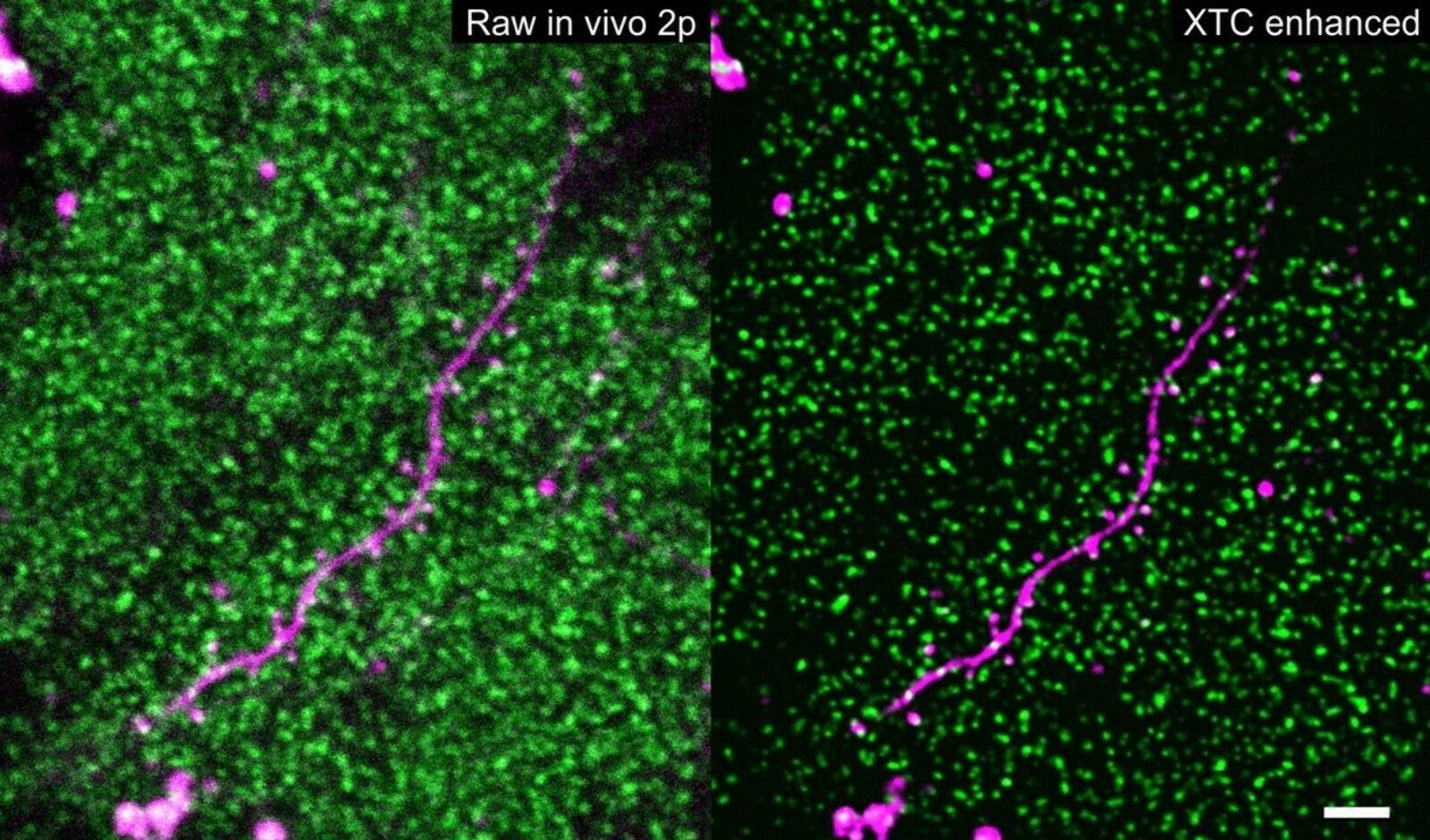
The Author
Connection points between neurons in the brain – called synapses – are hardly fixed and stable. They shape neuronal circuits by strengthening and weakening based on what a human or animal experiences. This phenomenon, called synaptic plasticity, is thought to be one of the molecular foundations that is important for learning. But one big goal has been to view the process of synaptic change in the living brain – this technological achievement has remained out of reach.
That type of evidence is important because it would enable researchers to directly connect specific molecular changes in neurons and changes to brain circuitry to an animal’s behaviors or experiences. Now, by combining state of the art in vivo imaging with machine learning, a team led by researchers at the Kavli Neuroscience Discovery Institute at The Johns Hopkins University (JHU) has accomplished the feat in mice. “Synapses are what guide the connections between neurons, and we can track them in vivo now,” says Adam Charles, an assistant professor of biomedical engineering at JHU, who co-led the work. “We don’t have to cut open the brain and put sections under a microscope – we can attach a microscope directly to the head and see how those connectivity patterns change in real time, at the resolution of a single synapse.”
The catalyst for this achievement was a neuroscience graduate student named Yu Kang “Tiger” Xu, says Jeremias Sulam, also an assistant professor of biomedical engineering at JHU who co-led the work. While studying in a lab focused on understanding how the brain wires itself through learning, Xu saw how powerful the ability to image individual synapses could potentially be.
Researchers can observe synaptic strength by genetically engineering mice in which proteins at synapses fluoresce, then the strength of the fluorescence signal can be tracked using light intensity as an indicator. In thin slices of brain tissue, sophisticated imaging techniques can reveal individual synapses. “But of course, then the animal is no longer living,” says Sulam, “so you can’t see how synapse strength changes,” as it learns to navigate a maze, for example, or encounters a frightening situation. One of the most powerful imaging methods that can be used in living animals, called two-photon microscopy, lacks the resolution to capture individual synapses. This type of microscopy would capture “just blobs and blur and overall of brightness,” he says.
Xu and his computational mentors, Sulam and Charles, wondered whether modern machine learning algorithms, which excel at pulling a signal out of extremely noisy data, could get around this challenge. “If you have a bunch of examples of inputs to your system, and a bunch of corresponding examples of how those should look like, these algorithms are really good at restoring them and producing high-quality outputs,” says Sulam. The researchers first created a set of high-resolution images of synapses in brain slices. Then they did something a little bit tricky: They created a set of low-resolution images of synapses in the brain slices too, using a microscopy technique with similar resolution and characteristics to two-photon imaging. Finally, they used the low-resolution images as inputs and the high-resolution images as outputs to train a deep neural network.
“It was a leap of faith in the beginning, we truly didn’t know if this was feasible at all,” says Sulam. But to everybody’s surprise, “after careful validation of our results, we saw that it actually works really, really well.” Glimpsing thousands of synapses that correspond to an animal experiencing the world was “really, really impressive,” he adds. “It’s the first time anyone has done this because no one was able to image individual synapses before.”
The new technique will allow researchers to study in unprecedented detail how the brain rewires itself under different circumstances, the researchers say – including normal learning that occurs during development, negative experiences at the root of psychiatric conditions such as post-traumatic stress disorder, and disease processes in which brain circuitry goes awry, such as Alzheimer’s disease. It may even reveal changes in synaptic strength that occur under conditions that researchers often consider static – like when a mouse is simply exploring its own, familiar home cage – and those revelations might in turn inform and add nuance to computational models of brain function. “We really think this is a useful, viable tool that is going to just open up this area,” says Charles. “It will be really interesting to see where people take it – not just scientifically, but also technologically.”
Charles and Sulam are continuing to refine the approach. They also note that the same idea – creating a training set of degraded data and then training an algorithm to restore it – can potentially be used to obtain better detail in other types of in vivo fluorescent images, too. “People seem excited about the capabilities of this tool set,” says Charles.